The ability of processing crossmodal information is a fundamental feature of the brain that provides a robust
perceptual experience for an efficient interaction with the environment. Consequently, the integration of multisensory
information plays a crucial role in autonomous systems to create robust and meaningful representations of objects and events.
For dealing with real-world information, an autonomous, intelligent system must be capable of processing, integrating, and
segregating different modalities for the purpose of coherent perception, decision-making, and cognitive learning.
This half-day workshop will focus on presenting and discussing new findings, theories, systems, and trends in computational models of crossmodal learning. The goal of the workshop is to discuss different crossmodal learning mechanisms, models, and theory and how this can improve computational models. The workshop will feature a multidisciplinary list of invited speakers with outstanding experience in crossmodal learning. The main discussion will focus on how psychological and neurophysiological findings can be adapted to computational models.
The primary covered topics are (but not limited to):
Important dates: Paper submission deadline: August 11 2017 Notification of acceptance: August 25, 2017 Camera-ready version: September 05, 2017 Workshop: September 18, 2017
Selected contributions will be presented during the workshop as spotlight talks and in a poster session.
Contributors to the workshop will be invited to submit extended versions of the manuscripts to a special issue to be arranged. Submissions will be peer reviewed consistent with the journal practices.
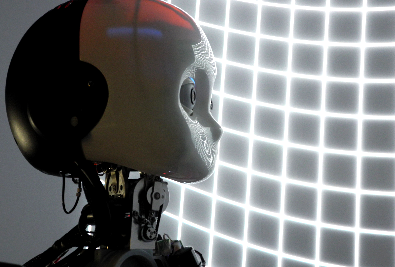
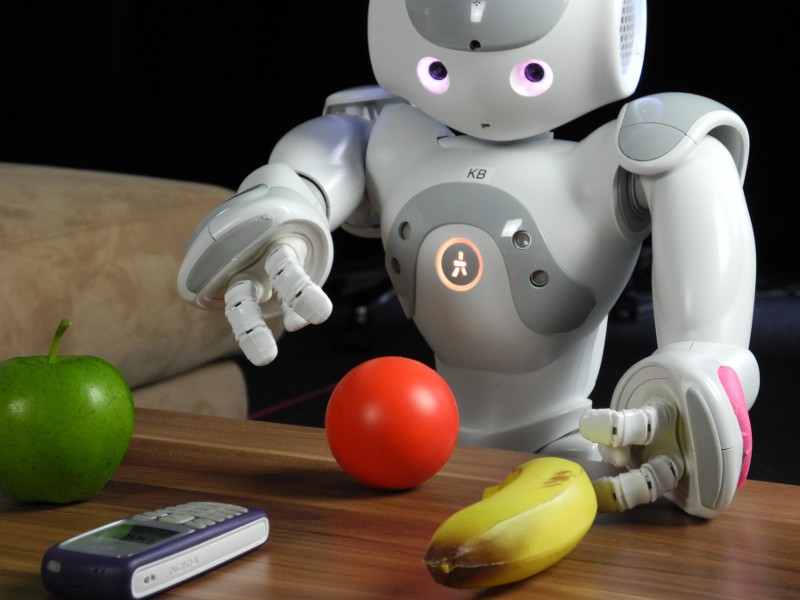
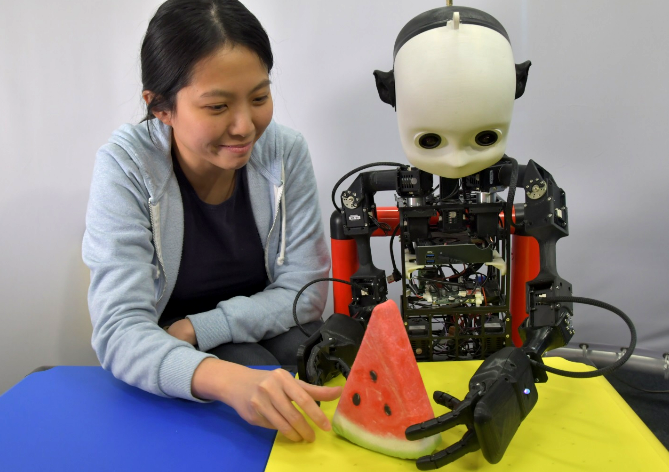
This half-day workshop will focus on presenting and discussing new findings, theories, systems, and trends in computational models of crossmodal learning. The goal of the workshop is to discuss different crossmodal learning mechanisms, models, and theory and how this can improve computational models. The workshop will feature a multidisciplinary list of invited speakers with outstanding experience in crossmodal learning. The main discussion will focus on how psychological and neurophysiological findings can be adapted to computational models.
The primary covered topics are (but not limited to):
- Machine learning and neural networks for multimodal learning
- Behavioral studies on crossmodal learning
- Models of crossmodal attention and perception
- New theories and findings on crossmodal processing
- Bio-inspired approaches for multisensory integration
- Deep learning architectures for multimodal perception
- Multimodal systems for robotics.
Important dates: Paper submission deadline: August 11 2017 Notification of acceptance: August 25, 2017 Camera-ready version: September 05, 2017 Workshop: September 18, 2017
Selected contributions will be presented during the workshop as spotlight talks and in a poster session.
Contributors to the workshop will be invited to submit extended versions of the manuscripts to a special issue to be arranged. Submissions will be peer reviewed consistent with the journal practices.