Workshop on Bio-inspired Social Robot Learning in Home Scenarios
Sponsored by TC-CoRo
October 10th - Daejeon, Korea
There has been considerable progress in robotics in the last years allowing robots to successfully contribute to our society. We can find them from industrial environments, where they are nowadays established, to domestic places, where their presence is steadily rising.
This workshop explores the following question: "How well prepared are learning robots to be social actors in daily-life home environments in the near future?".
This workshop is therefore not only an opportunity to address this focus on the latest scientific contributions on bio-inspired learning social robotics, but also links this with the presence of robots in people daily-life environment. Thus, the main goal of this workshop is offering a common space for roboticists from different fields of expertise to discuss the current state-of-the-art of learning methods in robotics specially applied to home scenarios and recent developments in assistive robots.
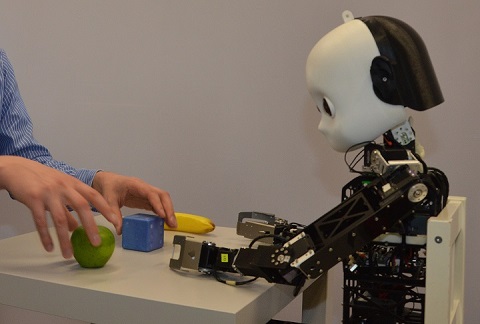
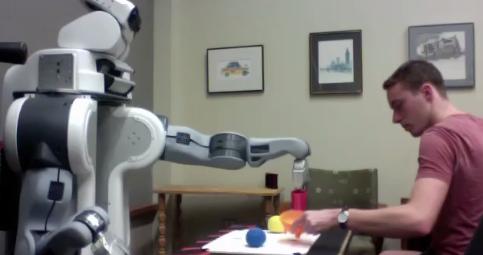
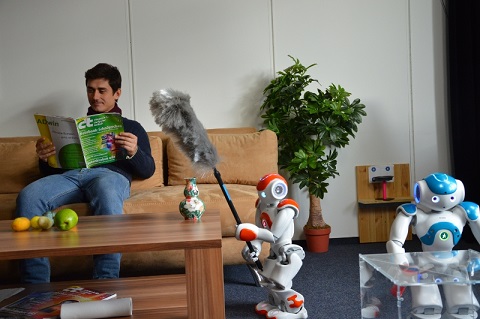
Topics of interest in this workshop
Roboticists are aware of the big challenges that involve working with service and assistive robots in home environments to develop real robot domestic applications. For instance, the RoboCup initiative founded a specific league "RoboCup@Home league" to aim the development of highly interactive intelligent robots to perform tasks in new and complex environments while being able to anticipate and resolve conflictual situations that may lead to mistakes or incomplete performance.
Such complex learning tasks in home environments can include among others learning to:
- Provide help in home services,
- Wipe/tidy up a table, floor, or room,
- Cook a meal,
- Be of assistance for elderly people,
- Be a conversational companion.
- Interactive reinforcement learning,
- Neural sequence learning
- Policy and reward shaping,
- Learning of object affordances and contextual affordances,
- Predictive learning from sensorimotor information,
- Learning understanding of environment ambiguity,
- Learning with hierarchical and deep neural architectures,
- Bootstrapping complex action learning in robots,
- Learning supported by external trainers by demonstration and imitation,
- Parental scaffolding as a bootstrapping method for learning.